Why do we need to index? It is for Improve query performance and making reading faster. That’s about it! But why, really? Slow queries are one of the most (if not the most) common causes of poor application performance. A slow query can be the difference between a site being super snappy and being literally unusable. Sometimes the difference between these two extremes is just one good index away. So how does database indexing work?
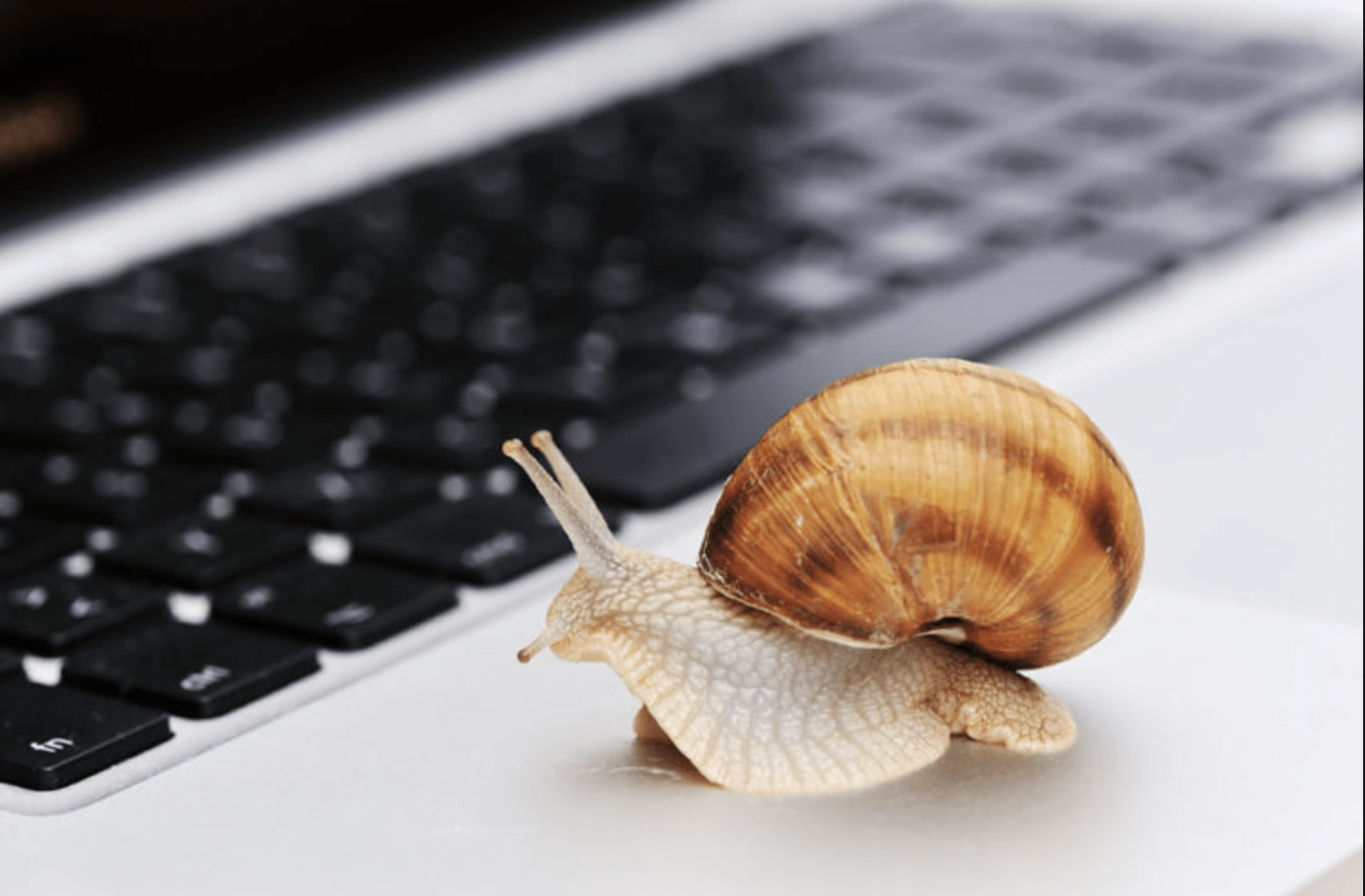
Indexing is a lot more than just throwing an index at every column in our WHERE clause and hoping that one of them sticks. If we don’t know what we are doing, a bad index can actually make performance worse.
Let’s find out how much they actually are to this topic.
What Is an Index?
Let’s take an example of a phone book. For simplicity’s sake, let’s say it has three pieces of information: First name, last name, and phone number. A phone book is almost always going to be ordered by the last name. So if we’re given someone’s last name, we can find that person pretty quickly because the phone book is ordered for exactly that type of search query.
If we’re only given someone’s first name, then we have to look through every single entry and we will probably end up with a whole bunch of results. We could say that this phone book is having an index on the last name. An index is an ordered representation of the indexed data.
Question: Why is order so important?
What we’re doing when we’re querying our data is we’re searching our data for a subset of that data. It turns out that searching for an ordered input is a lot more efficient than searching for an unordered input. So what is the index actually? That’s where the B-Tree structure comes from.
Recommended reading: Why is it needed for indexing!
How Does Database Indexing Work on B-Tree?
1. What is B-Tree?
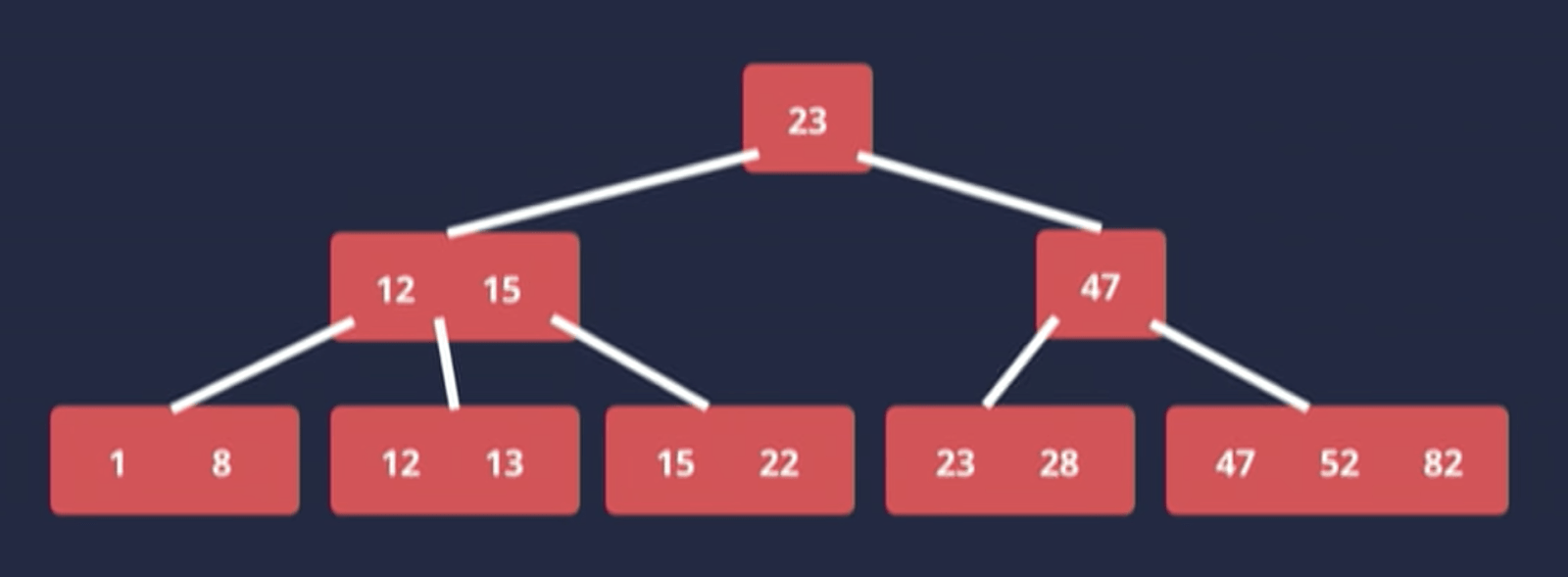
From the example above, the root node is 23. We have the left subtree and the right subtree. All the values in the left subtree are less than 23 and all the values in the right subtree are greater than or equal to 23. This is true for every node in the tree. This is how the tree is ordered. The nodes at the very bottom are called the leaf nodes, they don’t have any further subtrees and they are all in the same level or the same depth. That’s why we call it B-Tree, B stands for Balance.
Recommended reading: What Is Data Classification: Best Practices And Data Types
2. How does it work?
This is important because this way we can guarantee that it takes the same number of steps to find any node/value in the tree. If we have one set of the tree that is way deeper and unbalanced then searching for a value in that part of the tree will take longer, which is not what we want.
The leaf nodes will always be at the same level. They also form a doubly-linked list (indicated by 2 arrows pointing back and forth). So each set of leaf nodes has a pointer to the next set of leaf nodes and also to the previous set.
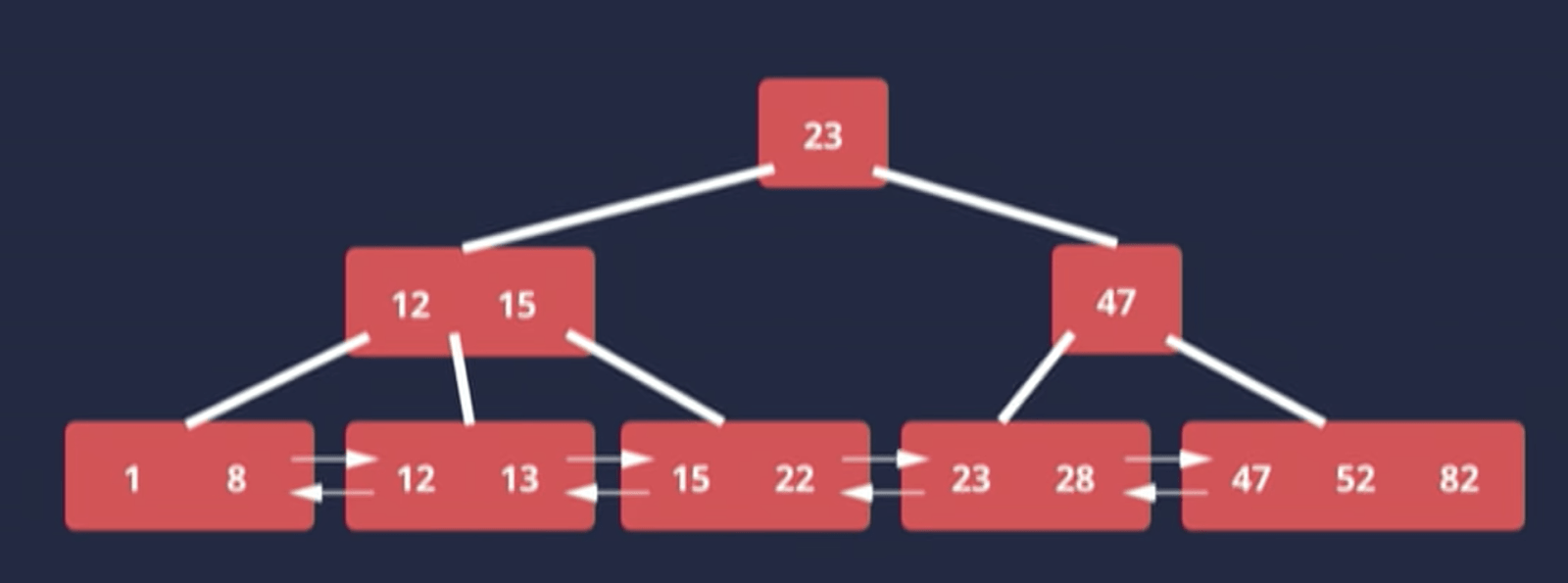
3. Why is that important?
When we’re searching for data in an index, we are going to spend a lot of our time down in those leaf nodes scanning through them. If we don’t have a doubly-linked list then as soon as we hit the end of one of those leaf nodes, we would have to go back up the tree, find the next leaf node, start scanning and go up again. That’s not very efficient. By having a doubly-linked list, we can just scan through them sequentially without having to back up the tree every time.
4. What data is actually stored on the index?
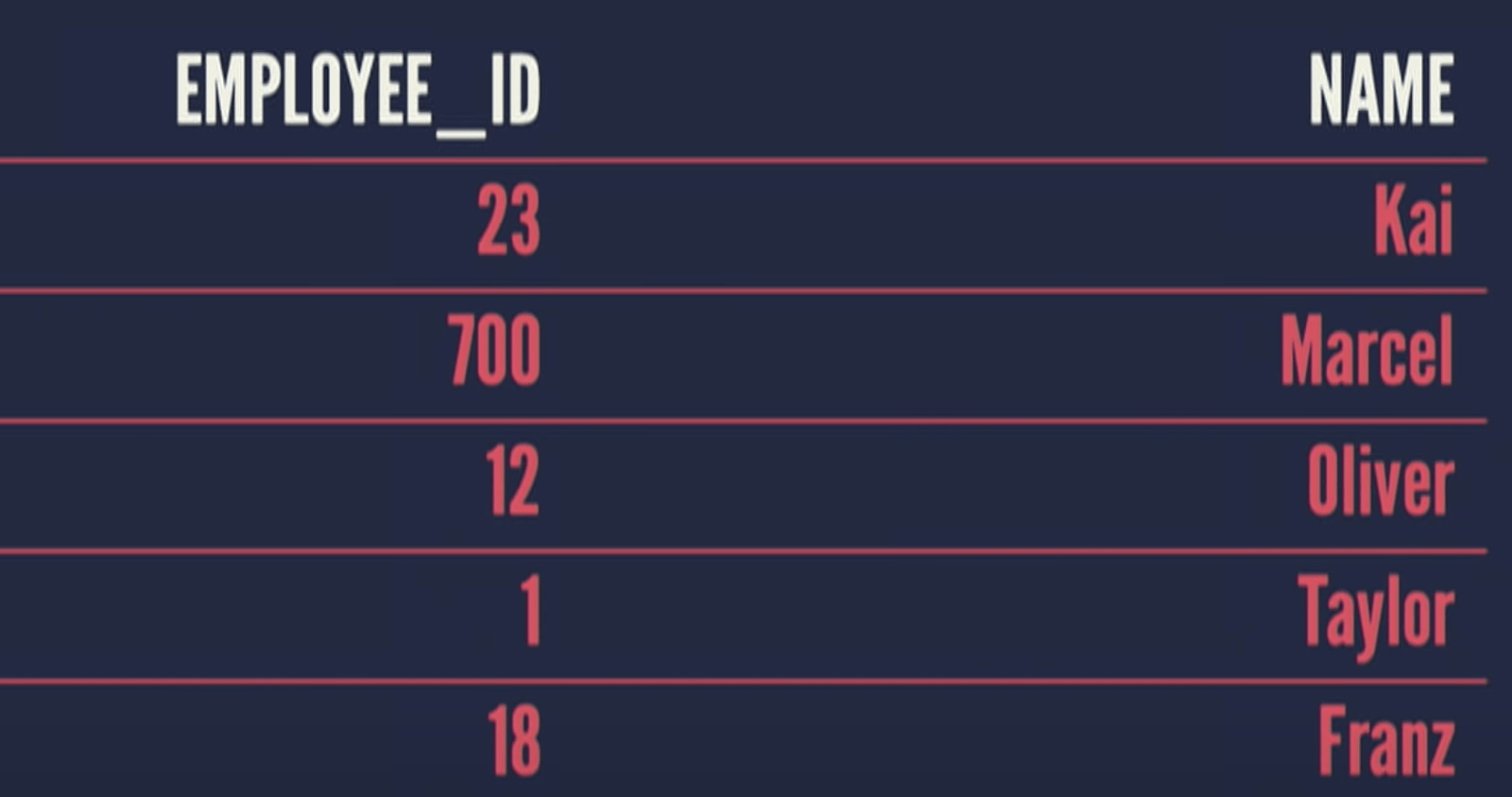
Let’s say we have a simple table with employee_id and name. If we put an index on the name column, the index would end up looking like this
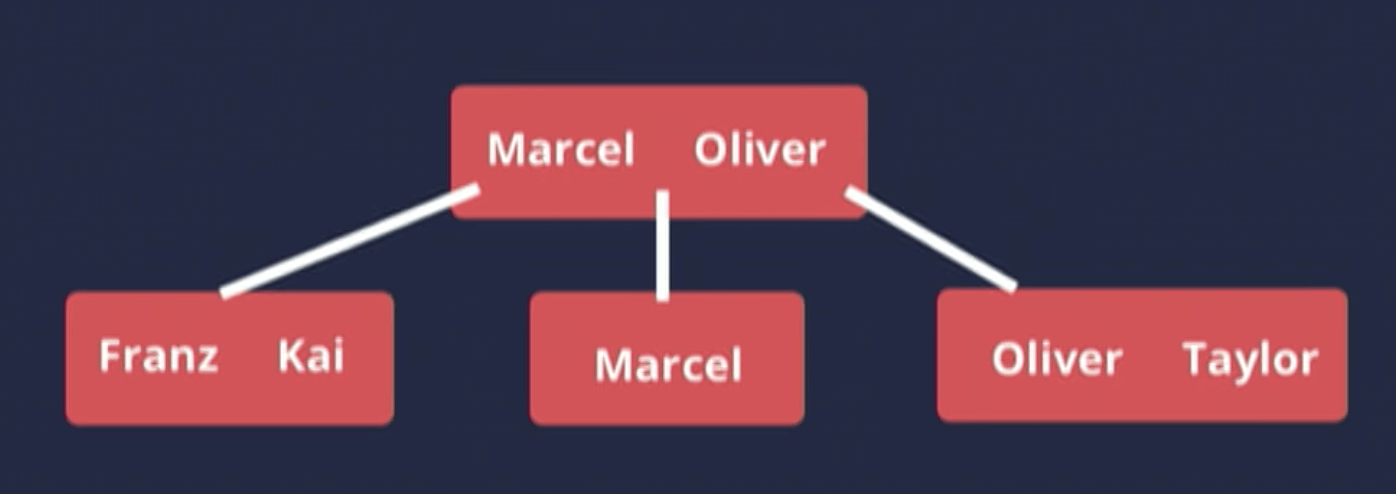
The employee_id is nowhere to be found on this index, it only contains the name. In other words, an index only contains the values of the column we actually put the index on.
5. For more detail
Question: If we need data that is not on the index, how do we get them? In this case, how could we also get the employee_id?
The above image is actually incomplete. The fact is that the database stores one additional piece of information on the index for every value. It’s called Row ID.
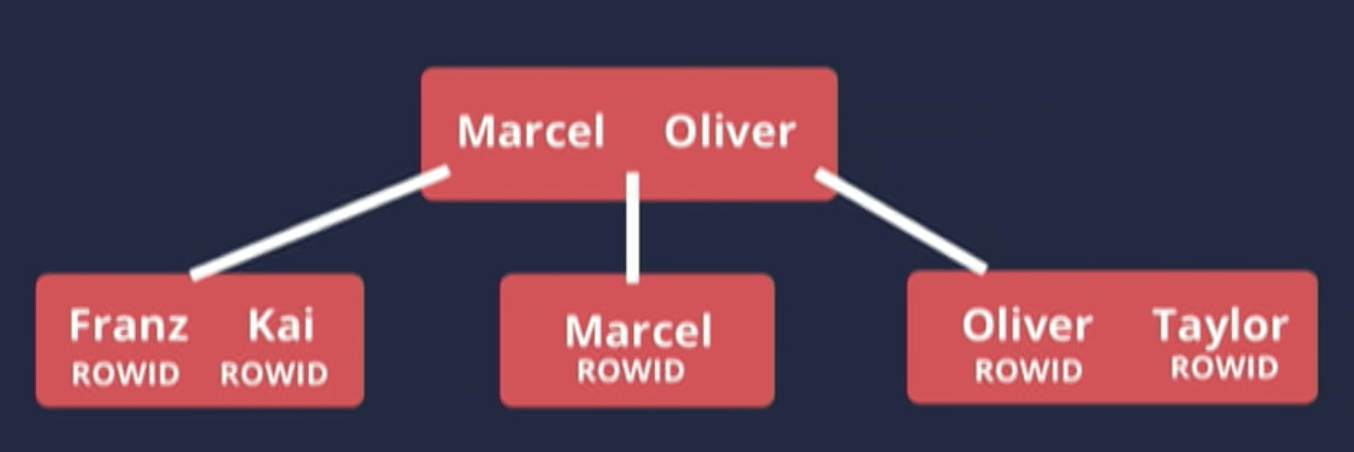
It’s a database internal identifier that identifies a particular row in a table (it’s not our primary key). That way we can go back to the table and find the corresponding row to which this value in the index belongs.
Question: What are the consequences of choosing that B-tree data structure?
- Searching for a value is very fast because of the binary search algorithm
- Logarithmic scalability. It doesn’t really matter how large our input gets, it can still keep performing.
Question: If an index makes our queries faster then why don’t we put an index on every single column?
Recommended reading: How to Increase the Quality of Your Data
How Does Database Indexing Work on Other Structures?
Using an index improves read time but it makes writing slower. With every insert, update and delete we perform, we also have to insert, update and delete the index which would mean the index will have to be potentially rebalanced (which will take time). If we have a whole bunch of indexes, our Writes are going to be very slow. So the rule is, that we want to have as many indexes as necessary but as few as possible.
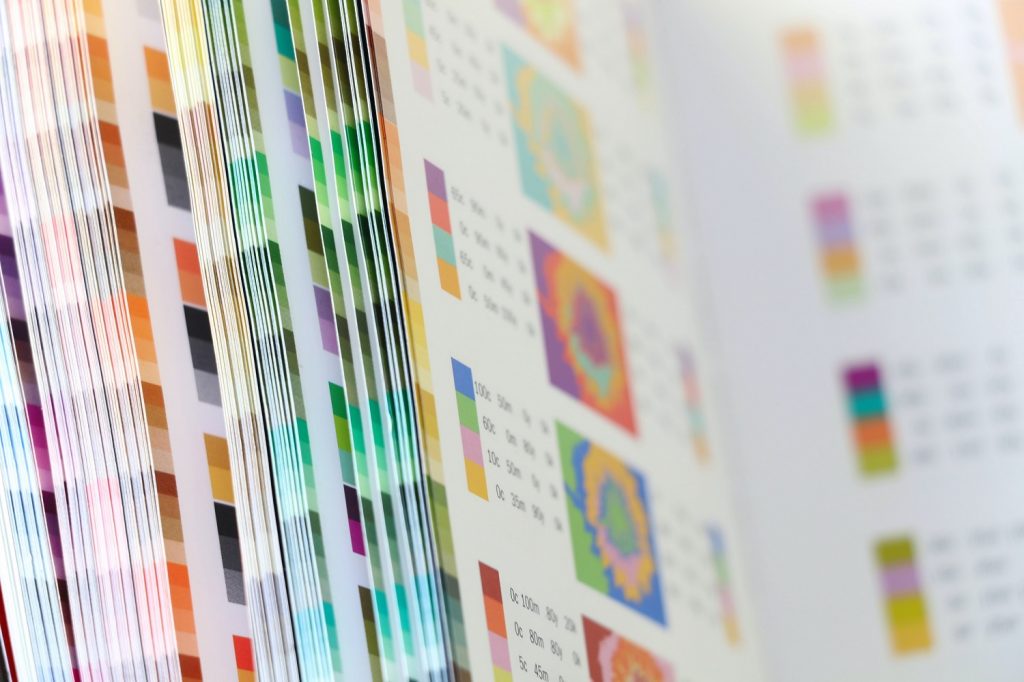
Understanding the database’s execution plan can result in writing better queries, better indexing, and better application performance, and ultimately it could help us to become a better developer when it comes to working with databases.
Question: What is the execution plan?
Answer: It’s the steps that our database needs to perform/execute our query.
Question: How do we get an execution plan?
Answer: Almost for all database vendors, we just have to prepend an EXPLAIN to our query.
For example: Instead of writing SELECT * FROM users WHERE id = 1, we use EXPLAIN SELECT * FROM users WHERE id = 1. On MySQL, we will get a result that looks something like this:
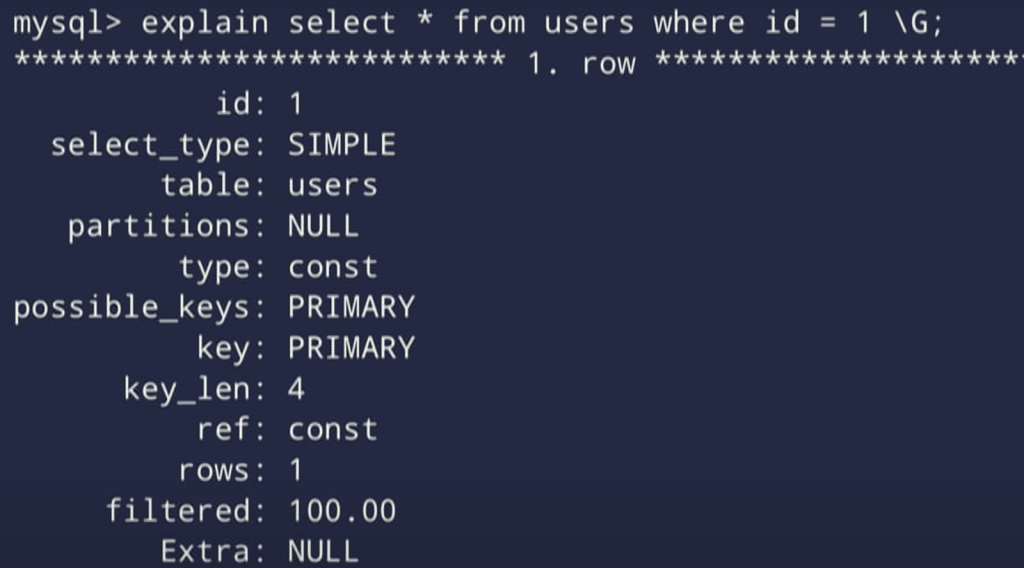
We’re not going to look through all of them, but we will look at the most important one which is the Type column. In the picture, it said “const”. On the Mysql documentation, it will be called the ‘join types’. It’s a bit confusing but we can simply think of it as access types for simplicity’s sake. Because what it really tells us is how the database is going to access our data or how exactly it is going to use an index or not use an index to execute our query.
Let’s go to some possible values we might encounter in the explained output in that column and go through some characteristics of each one of them.
1. Const / eq_ref
These two values are actually distinct values. They might appear on their own in the EXPLAIN output, but because they behave in the same way so we could group them together and treat them as one.
The database will perform a B-Tree traversal to find a single value in the index tree. Basically, we’re doing a binary search. This can only get used if we can somehow guarantee the uniqueness of our result set (Fun Fact: LIMIT does not guarantee uniqueness, it just fetches the data and discards the afterward).
That means there can be at most one result. Two ways we can do this:
- Have a primary on the column
- Have a unique constraint on the column
Basically, we’re starting at the top of the tree and then we go left or right depending on if the value is less than or greater. Until we either find the value or find out that there is no value. It is super fast. If we see Const or Eq_ref in the EXPLAIN output, then we can stop optimizing because it will not get any faster.
2. Ref / Range
Again they are two distinct values that get grouped together because of their similar behavior. They are known as index range scans. They also perform a B-tree traversal, but instead of finding a single value, they find the starting point of a range, and then they can from that point on. Let’s say we have a query “WHERE id > 15 AND id < 30”.
The database will do a traversal to find the first value (which is greater than 15). From that point on, it will start scanning through the leaf nodes (doubly linked list) until it hits the first value that is greater than or equal to 30. These rows are the only rows the database has to look at.
So the ref/range limits the total number of rows the database has to inspect to perform our query. It’s a good thing because of less work for the database. It stops if it finds a value that does not meet the criteria.
3. Index
Also known as a Full index scan. We’re still using the index but we’re not using it to limit the number of rows. We literally start at the very first leaf node and scan through all of them until we hit the very last leaf node. There is no filtering of anything going on, we simply traverse through the entire index (but we’re still using the index).
4. All
Also known as a Full table scan. It’s as bad as it sounds, we should avoid it at all costs, it’s never what we want. It doesn’t use an index at all. It loads every column of every row from the table into memory, goes through them one by one, and then omits or discards them depending on whether or not they fulfill the filter criteria. In other words, it scans through all of them and emits or discards accordingly.
5. Recap
Access types:
- Const /eq_ref: Basically binary search to find a single value, stops if we got that. It’s super-fast, almost perfect.
- Ref/range: We use the index to limit the number of rows to a certain subset of rows that we even have to look at
- Index: Use the index but not for limiting or filtering, we’re just scanning through every value in the index
- All: Avoid it at all costs, we just load up everything and go through it.
The Common Pitfalls
1. Functions
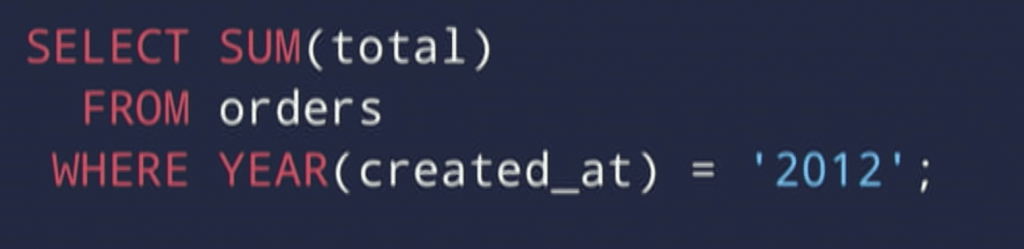
If we have a query like this, it doesn’t matter what goes into to function because the column will not be seen by the database at all. This is because we can not guarantee that the output of the function has anything to do with the index values.
2. Inequality operators

If we run that query, we can see that our database still has to look through a lot of rows in our database even though we have already put an index on these two columns (created_at and user_id).
Why is that? The reason is that we have an inequality operation (BETWEEN) on the created_at column (the created_at column is our first index). It seems like the index just stops there.
In this situation, we can just simply put the user_id into the first position because we have an equality operation on user_id.
We at Designveloper hope with this article you can understand exactly how does database indexing work!
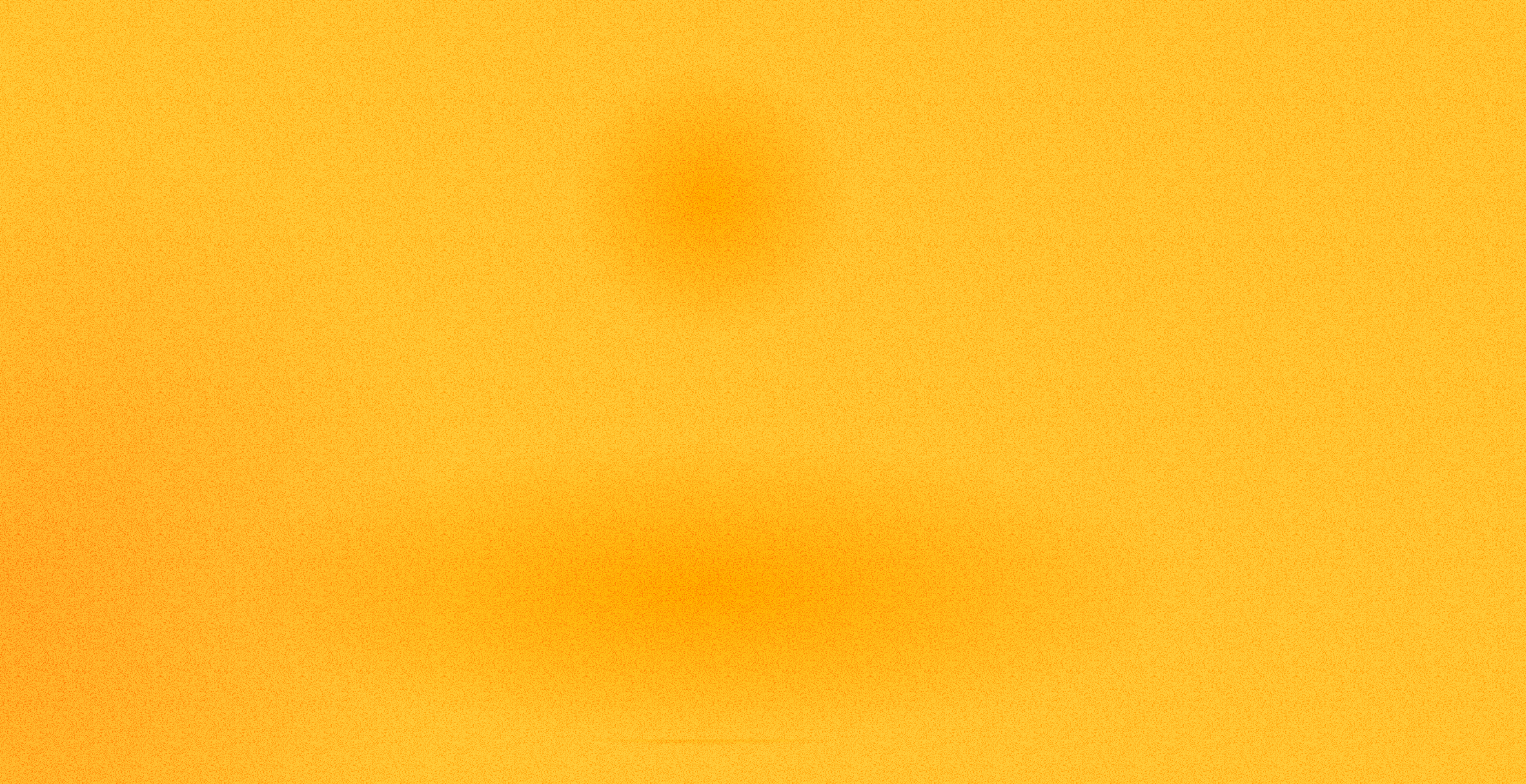
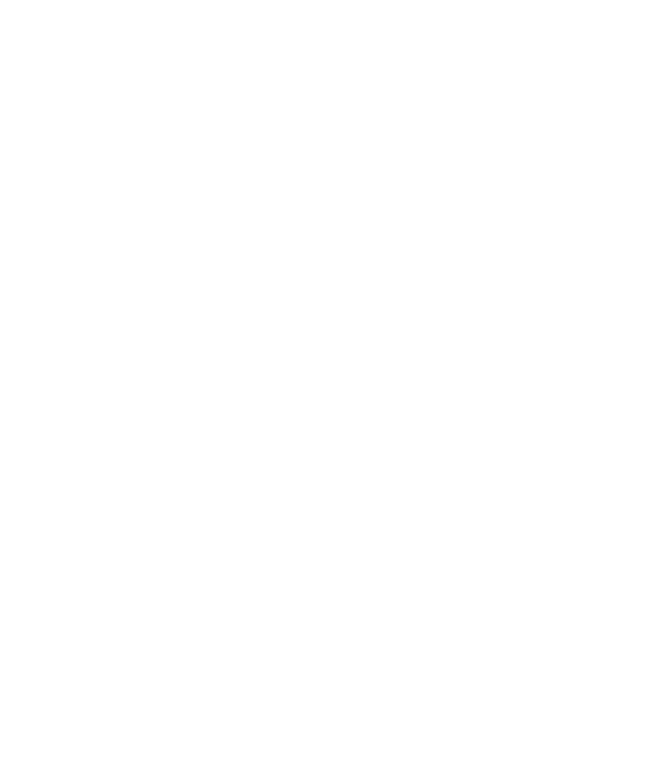
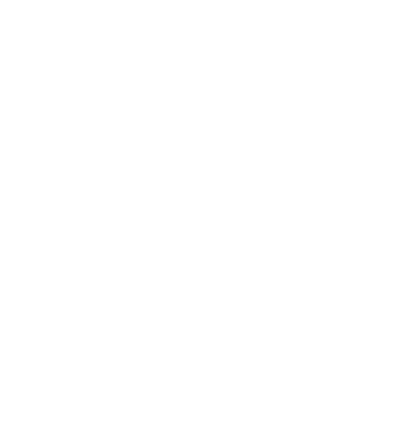
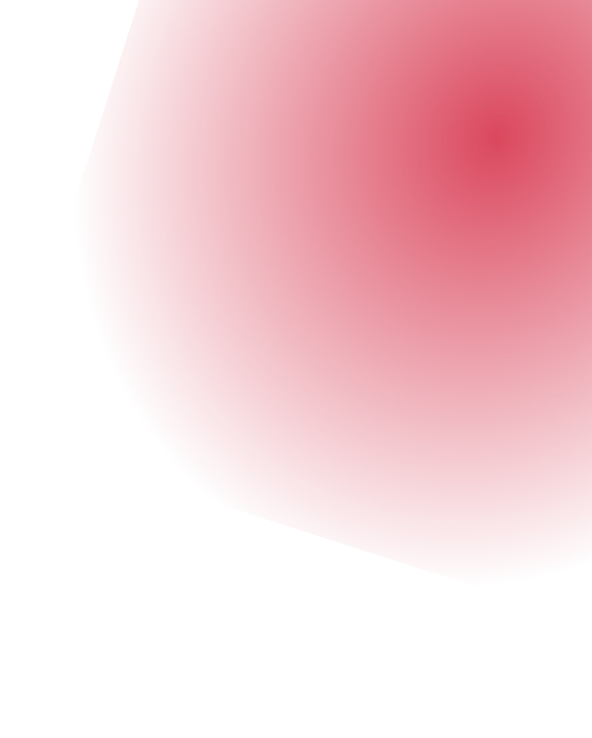
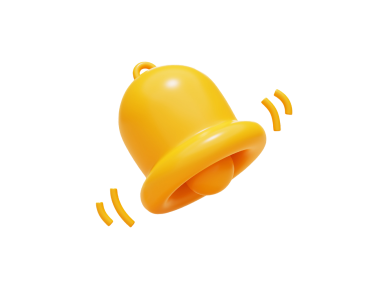
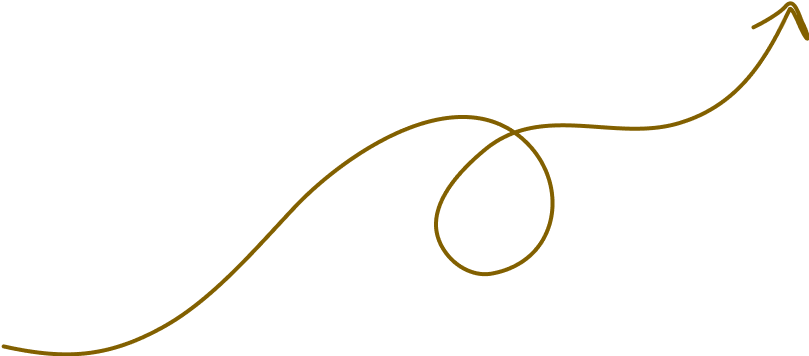
Read more topics
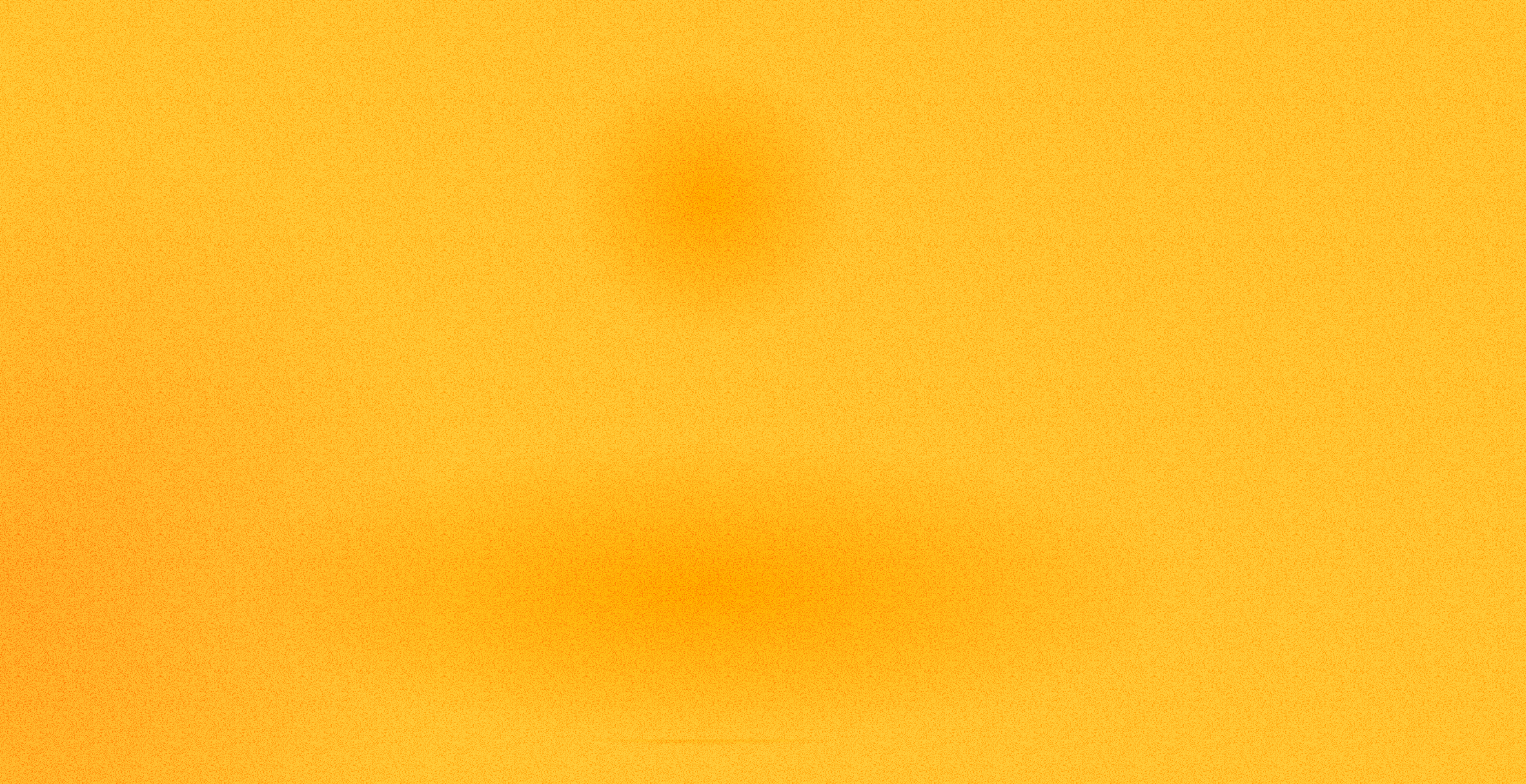
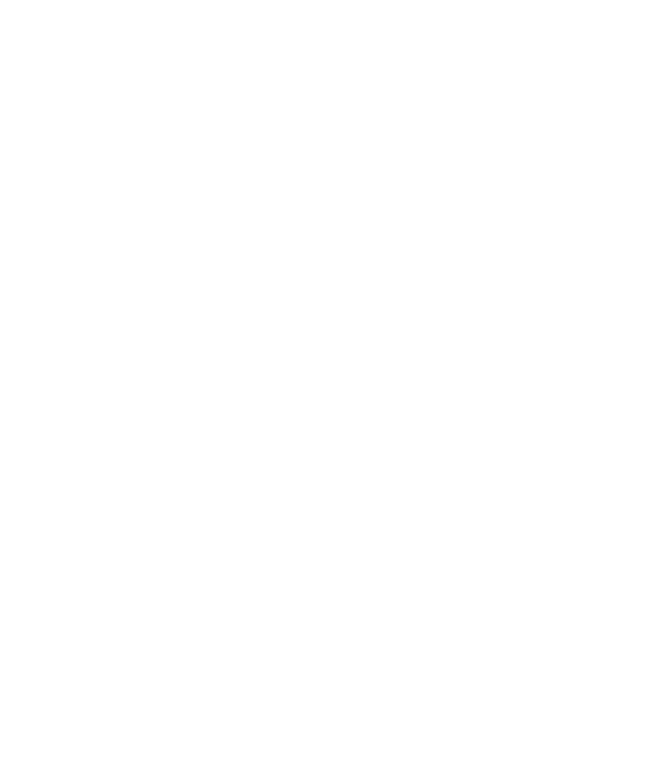
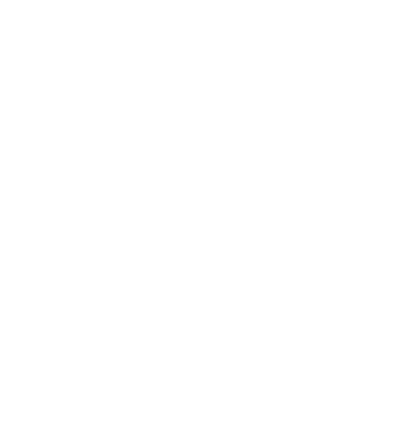
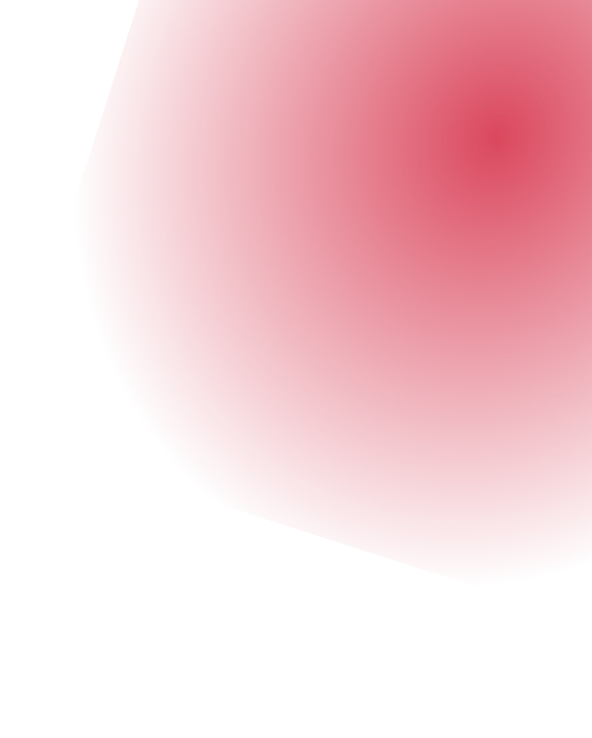
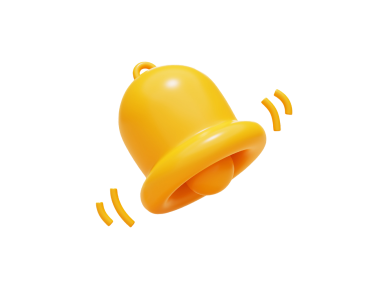
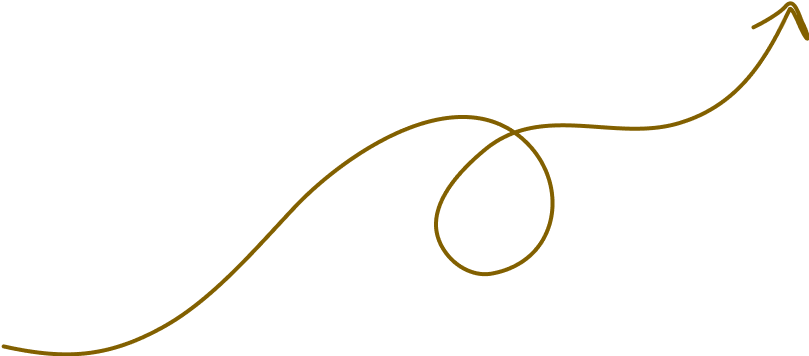